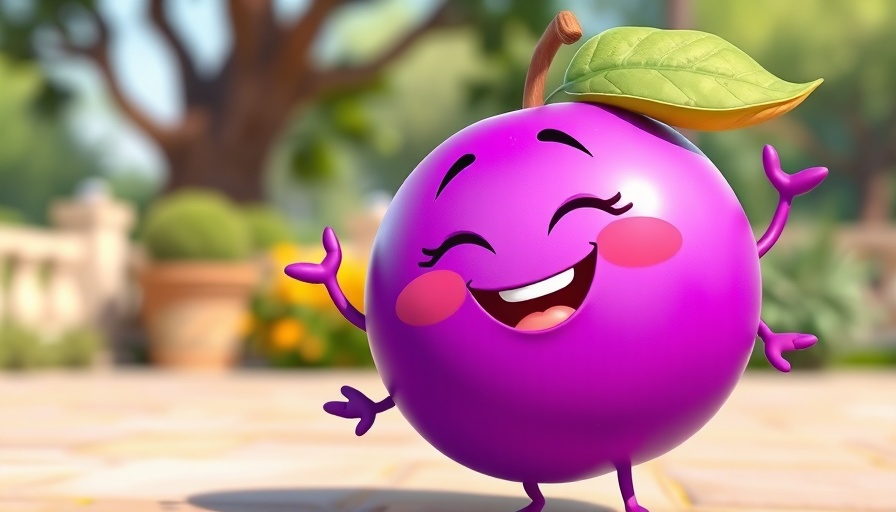
Pruna AI's Open Source Framework Transforms AI Model Optimization
Pruna AI, an innovative European startup specializing in AI model compression algorithms, has officially open-sourced its optimization framework. This useful tool integrates various efficiency techniques such as caching, pruning, quantization, and distillation to enhance AI model performance. Co-founder and CTO John Rachwan emphasized the comprehensive nature of their framework, which not only facilitates efficient model compression but also evaluates potential quality loss, ensuring users are informed about any trade-offs they make during the optimization process.
Significance of Comprehensive Optimization Tools
Rachwan notes that most large AI companies, including OpenAI, typically develop in-house solutions for model optimization. While various open-source tools exist, they often focus on single methods, lacking the integrative approach that Pruna is championing. By providing a one-stop solution, Pruna AI simplifies complex operations into a user-friendly format that enhances the usability of AI models across sectors. This comprehensive integration could prove invaluable, particularly in healthcare settings where accessibility and efficiency are paramount for delivering critical services.
The Promise of Advanced Features
Moreover, Pruna AI is set to roll out a 'compression agent,' which will automate the optimization process for developers. Users can simply specify their desired performance metrics—such as needing increased speed without significant accuracy loss—and the agent will find the best optimization methods, making AI model handling less resource-intensive and potentially cheaper for businesses. This automated feature is particularly advantageous for healthcare providers who rely on efficient, reliable technology.
Implications for Healthcare Innovation
As the healthcare industry increasingly incorporates AI to improve patient care through predictive analytics and diagnostics, tools like Pruna AI's framework can facilitate faster, more efficient data processing in clinical settings. By enabling healthcare IT professionals to quickly adapt AI models to meet specific needs and optimization requirements, this framework could help streamline operations, thereby improving patient outcomes and institutional efficiencies.
Write A Comment